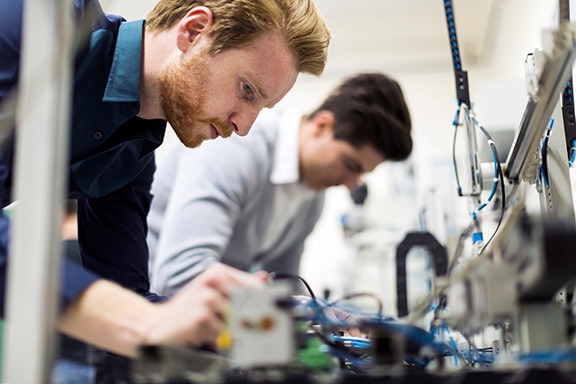
Discover the Perfect Product
Utilize our tool to identify the ideal onsemi product for your specific application with maximum efficiency.
Find Now
ソリューション
By Technology
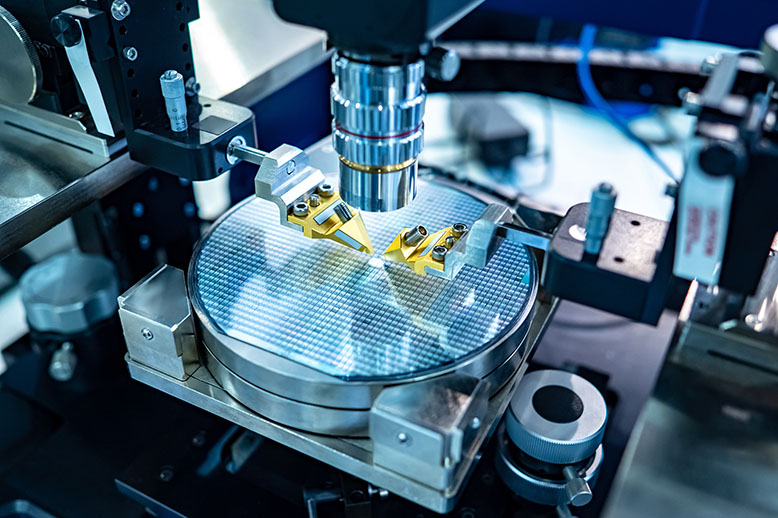
System Solution Guides
Delve deeper with our user-friendly system solution guides, invaluable resources crafted to elucidate onsemi's diverse product range across various applications.
Explore Guides
デザイン
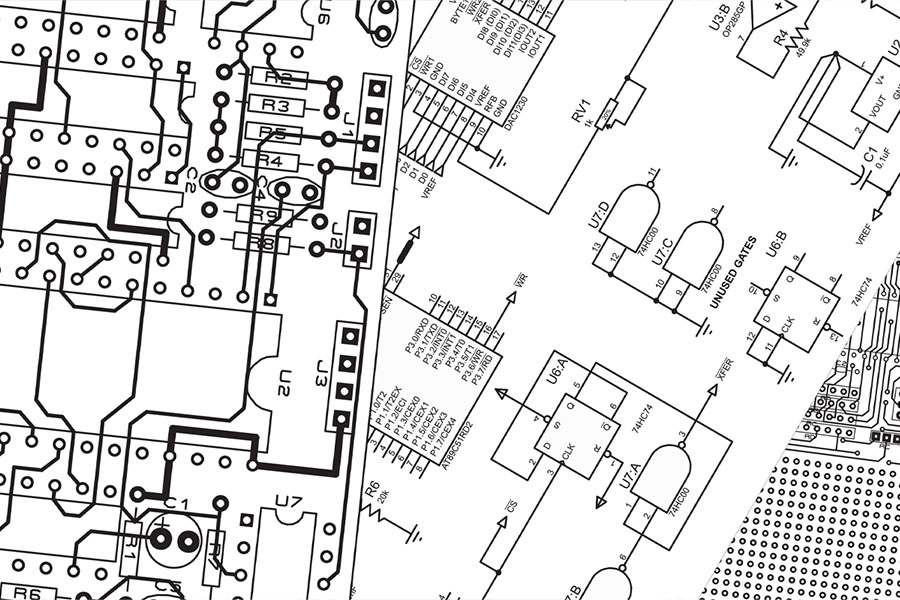
Interactive Block Diagrams
IBD provides a detailed and organized overview of the technology's design, relevant product information and specification.
Explore Diagrams
会社概要
Environmental, Social and Governance
Governance Documents年次サステナビリティレポート社会的責任多様性、公平性、包括性Giving Now プログラム
Ethics & Compliance
How to Report a Concern or IncidentCode of Business ConductProtection from Retaliation
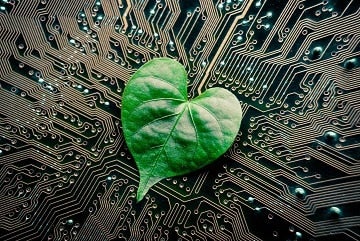
年次サステナビリティレポート
オンセミは、よりよい未来を築くための破壊的イノベーションを推進しています。2023年のサステナビリティレポートでは、環境、社会、ガバナンスのイニシアチブに関する取り組みについて詳しく説明しています。
レポートを見る